The electric vehicle (EV) industry has grown rapidly over the past decade, with more and more drivers choosing to switch from traditional gasoline-powered vehicles to electric ones. As the EV industry continues to expand, the issue of ensuring accurate predictions of state-of-charge (SOC) of electric vehicles while charging has become increasingly important. Accurate predictions of SOC are critical for EV drivers, as they rely on these predictions to plan their trips and ensure they have enough power to reach their destination.
In order to accurately predict the SOC of an EV, it is important to understand the factors that can affect the SOC. These factors include the size of the battery, the type of charger used, the charging profile, and the temperature of the battery. Additionally, the amount of time taken to charge the EV is also an important factor in predicting the SOC.
To accurately predict the SOC of an EV while charging, a variety of methods have been proposed. These methods range from simple linear regression models to more complex artificial neural networks. The accuracy of these methods can be further improved by incorporating real-time data and battery information into the prediction models. Additionally, the use of smart chargers that can adjust the charging rate based on the SOC can also help improve the accuracy of SOC predictions.
The accuracy of SOC predictions is critical for EV drivers, as it allows them to plan their trips and ensure their vehicles have enough power to reach their destination. By understanding the factors that affect the SOC of an EV and using advanced prediction models, the accuracy of SOC predictions can be improved.
Factors Affecting SOC Prediction Accuracy
The accuracy of the State of Charge (SOC) prediction of an electric vehicle (EV) is affected by several factors. These include battery characteristics such as capacity, temperature, and current; the type of prediction method used; and the accuracy of the predictive algorithms and models. Battery capacity affects the amount of energy stored in the battery, and thus how accurately the SOC can be predicted. Battery temperature affects the rate of charging and discharging of the battery, which can lead to inaccurate SOC predictions. Battery current affects the rate of battery aging, which can also lead to inaccurate predictions.
The type of prediction method used also affects the accuracy of SOC predictions. A model-based method uses mathematical models of the battery and its characteristics to predict the SOC. This type of method is more accurate than other methods, though it is more complex and requires more data. A data-driven method uses historical data from the battery to predict the SOC. This method is less accurate than model-based methods, but it is simpler and requires less data.
Accurate predictive algorithms and models are also necessary for accurate SOC predictions. Predictive algorithms can use data from the battery to accurately predict the SOC. Models can also be used to predict the SOC by considering the battery’s characteristics and behavior. Both algorithms and models must be accurate in order for the SOC to be accurately predicted.
In conclusion, the accuracy of the SOC of an electric vehicle can be accurately predicted if the battery characteristics, type of prediction method, and accuracy of the predictive algorithms and models are taken into account. By taking these factors into consideration, the SOC of an electric vehicle can be accurately predicted while charging.
Types of SOC Prediction Methods
The type of SOC prediction method used can have a significant impact on the accuracy of the prediction. Generally, the two primary SOC prediction methods are model-based and state-of-charge estimation. Model-based methods are based on physical models of the battery and its components, and they can provide accurate predictions over different operating conditions. State-of-charge estimation methods use measured data from the battery and its components to estimate the SOC of the battery. These methods are more tolerant of different operating conditions, but they can be less accurate than model-based methods in some cases.
When it comes to electric vehicles, SOC prediction is especially important because it allows the driver to know how much charge is left in the battery and how far they can travel before needing to recharge. The accuracy of the SOC prediction can be improved by choosing the right prediction method and making sure that all of the necessary data is collected and processed correctly.
Can the SOC of an electric vehicle be accurately predicted while charging? The accuracy of the prediction depends on the type of SOC prediction method used. Model-based methods are generally more accurate than state-of-charge estimation methods. However, both methods can be affected by external factors such as temperature and driving conditions. Therefore, the accuracy of the SOC prediction can be improved by carefully choosing the prediction method and making sure that all of the necessary data is collected and processed correctly.
Battery Characteristics and their Impact on SOC Prediction
The state of charge (SOC) of an electric vehicle is an important factor that needs to be monitored and accurately predicted while charging. Battery characteristics such as capacity, aging, temperature, and resistance are some of the most critical parameters that affect the accuracy of SOC prediction. The capacity of a battery is a measure of how much energy it can store and is determined by the number of charge/discharge cycles it can go through. As the battery is used, its capacity diminishes over time which can lead to inaccurate SOC predictions. Temperature is also a key factor in SOC prediction accuracy, as the rate at which the battery charges or discharges is directly affected by its temperature. High temperatures can cause the battery to overcharge, leading to inaccurate SOC predictions. Lastly, electrical resistance can also affect the accuracy of SOC predictions. As the battery ages, its internal resistance increases, making it harder for the battery to charge and discharge and thus leading to inaccurate SOC predictions.
In summary, the accuracy of SOC prediction while charging an electric vehicle depends on several factors, such as battery capacity, aging, temperature, and resistance. By taking these factors into account, it is possible to achieve higher levels of accuracy in SOC predictions.
Predictive Algorithms and Modeling for SOC
Predictive algorithms and modeling for SOC (State of Charge) are essential components for accurately predicting the SOC of an electric vehicle. The most widely used algorithms are based on artificial neural networks, fuzzy logic, and support vector machines. These algorithms take into account various factors, including battery characteristics, driving conditions, and environmental conditions, which are used to determine the current and future SOC of the battery. In addition, these algorithms can be used to predict the remaining capacity of the battery, which is critical for ensuring the safety and reliability of the vehicle. Furthermore, predictive algorithms can be used to improve the efficiency of the charging process by optimizing the charging rate and duration. Finally, predictive algorithms can also be used to extend the range of the vehicle by accurately predicting the remaining capacity of the battery.
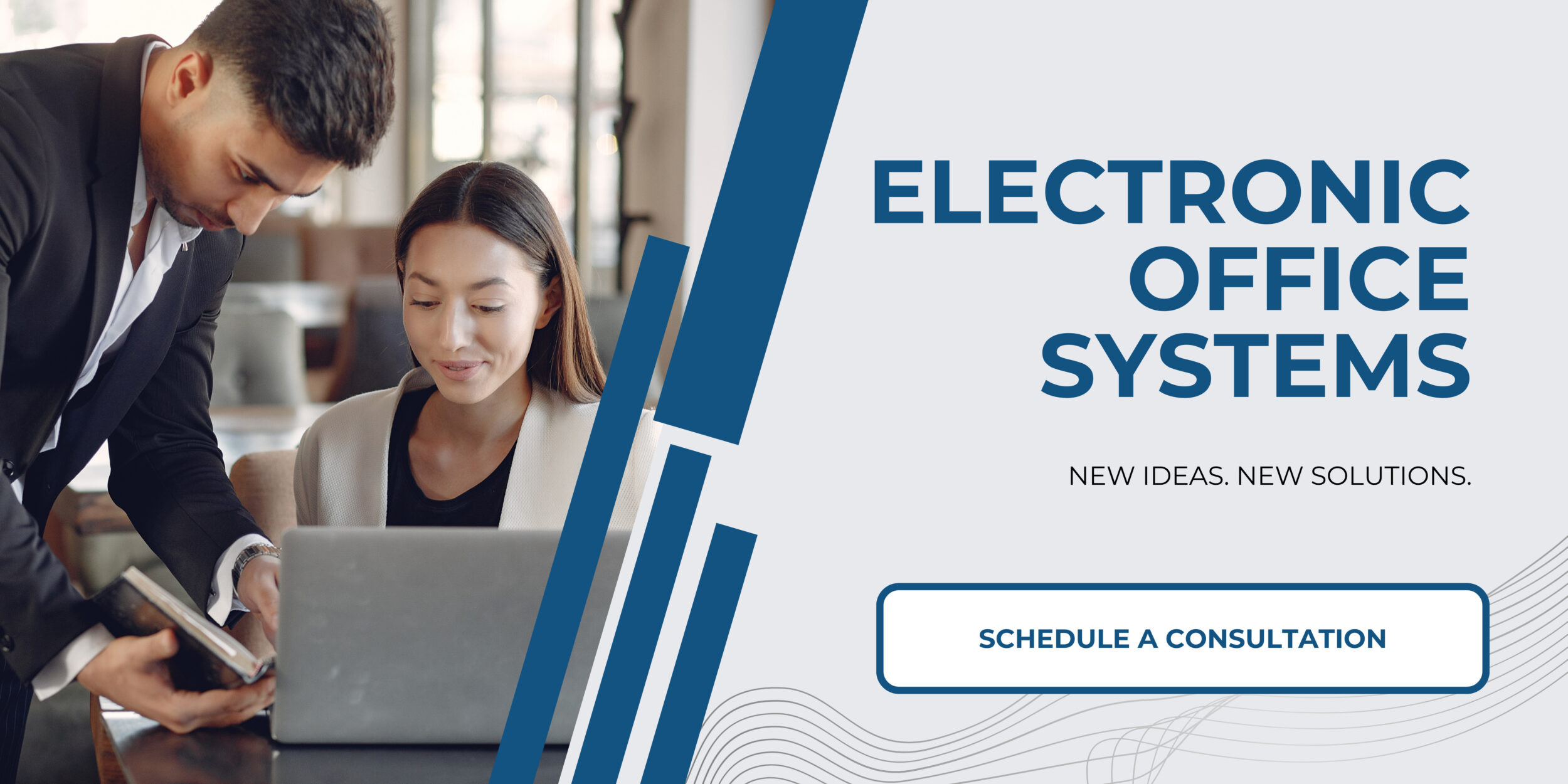
Challenges with Accurate SOC Prediction
Accurately predicting the state of charge (SOC) of an electric vehicle while charging poses several challenges. First, the charging infrastructure must be able to accurately read and report the current status of the battery. Additionally, the charging station must be able to accurately measure the current battery capacity in order to accurately predict the SOC. Furthermore, the charging station must be able to account for the varying battery characteristics, such as temperature, voltage, and resistance, which can affect the accuracy of the SOC prediction. Finally, the charging station must be able to account for the varying environmental conditions, such as humidity, wind, and temperature, which can also affect the accuracy of the SOC prediction. In order to achieve accurate SOC prediction while charging, these factors must be accounted for in the predictive algorithms used by the charging station.
In addition to the challenges posed by the battery characteristics and environmental conditions, SOC prediction accuracy can also be affected by the type of battery used. Lithium-ion batteries, for example, are highly sensitive to temperature, and the accuracy of SOC prediction can be adversely affected by extreme temperatures. Additionally, the rate of charge affects the accuracy of SOC prediction. If the rate of charge is too high, the accuracy of the prediction decreases. Furthermore, the accuracy of SOC prediction can be affected by the age of the battery, as older batteries can have different characteristics than new batteries and thus require different algorithms for accurate SOC prediction.
Finally, due to the complexity of predicting the SOC of an electric vehicle while charging, there are still some challenges that remain. For example, the accuracy of the predictive algorithms used by the charging station can be affected by factors such as the size of the battery, the type of battery, and the charging rate. Furthermore, the accuracy of the predictive algorithms can be affected by the test conditions used, such as the ambient temperature and the number of charging cycles. In addition, even if the predictive algorithms are accurate, the accuracy of the SOC prediction can still be affected by external factors, such as the vehicle’s driving conditions and the number of stops and starts.