In the realm of data analysis and information management, Intelligent Document Recognition (IDR) stands as a pivotal innovation that has revolutionized the way organizations handle vast volumes of unstructured data. IDR systems are designed to automate the process of extracting relevant information from a myriad of document formats, ranging from scanned paper documents to digital files in varying states of organization. This technology streamlines operations across multiple sectors by utilizing a blend of advanced methodologies such as machine learning, natural language processing, and optical character recognition. In this comprehensive article, we will delve into the mechanics of IDR, illustrating how it significantly bolsters efficiency in data extraction and document classification.
To comprehend the full impact of IDR, it is essential to consider the challenges it addresses. Before the advent of IDR, data extraction and classification were labor-intensive tasks prone to human error. Manual processing meant that key information might be missed, incorrectly entered, or inconsistently classified. IDR circumvents these issues by providing systems capable of learning and recognizing patterns within documents, reducing the margin of error and greatly accelerating the process.
This technology’s contribution to efficiency is multifaceted. First and foremost, IDR systems significantly reduce the time required to process documents. Automation enables near-instantaneous data extraction, freeing up employees for higher-value tasks. This increased speed comes with no sacrifice to accuracy; on the contrary, IDR often improves precision in data capture through consistent application of extraction rules, even across disparate document types.
In addition to accelerating the extraction process, IDR contributes to document classification in a manner that outstrips traditional methods. By leveraging machine learning algorithms, IDR systems can identify and categorize documents based on their content, layout, and even subtle contextual clues, a task that would be near-impossible for a human to perform with the same degree of consistency. This capability is crucial for enterprises that deal with large amounts of paperwork, such as legal firms, healthcare providers, and government institutions, ensuring that documents are sorted and accessible for future reference or analysis.
Lastly, the implementation of IDR goes hand-in-hand with increased data security and compliance. Automated classification and extraction mean sensitive information is less exposed to human touchpoints, reducing the risk of data breaches. Additionally, with programmed adherence to data handling regulations, organizations can ensure compliance with legal standards in a more streamlined and foolproof manner.
As we delve deeper into the technological intricacies and real-world applications of IDR, this article will underscore the significance of IDR in empowering businesses and organizations to harness their data more effectively, with a focus on the profound efficiencies brought about by intelligent document recognition technologies.
Advanced Pattern Recognition
Advanced Pattern Recognition (APR) refers to the capability of systems to identify complex patterns in data, which can then be used to classify data, recognize trends, predict behaviors, and make decisions. This technology underpins many of today’s sophisticated artificial intelligence and machine learning applications.
In the context of Intelligent Document Recognition (IDR), Advanced Pattern Recognition plays a critical role. IDR systems employ APR to scan through documents and extract meaningful information. Unlike simple pattern recognition, which might look for straightforward and repetitive patterns, advanced forms can handle more nuanced and irregular data. It can discern various types of document formats, handwriting, and even poorly structured data.
For example, APR systems can distinguish between a wide array of fonts and text sizes within a document, understand the context in which information is presented, and cope with variations in layout and design. They can interpret headers, footers, tables, and even text embedded in images. This level of understanding is essential for efficient data extraction because it reduces the need for manual preprocessing of documents and allows the IDR system to be more flexible and accurate.
When it comes to document classification, APR enables IDR systems to sort and categorize documents even when there are no explicit markers or identifiers. By recognizing patterns indicative of certain document types — such as invoices, legal contracts, or medical records — the IDR system can automatically classify incoming documents into predefined categories. This streamlines workflows, lessens the risk of human error, and saves an immense amount of time in document management processes.
Additionally, Advanced Pattern Recognition within IDR systems contributes to continuous learning. As the system encounters more documents, it can refine its recognition and classification models, thereby becoming more efficient over time. This inherent learning ability is part of what makes IDR systems powered by APR not only powerful at the outset but increasingly valuable as they adapt and improve through use.
In summary, Advanced Pattern Recognition is fundamental to the operation and success of Intelligent Document Recognition systems. It enables the sophisticated analysis of data necessary for effective data extraction and allows for the automated classification of documents into the correct categories with high accuracy. IDR systems that harness the power of APR are crucial for organizations that need to process vast amounts of unstructured or semi-structured data quickly and reliably.
Machine Learning Algorithms
Machine Learning Algorithms are the backbone of Intelligent Document Recognition (IDR). These algorithms allow systems to learn from data, identify patterns, and make decisions with minimal human intervention. Machine Learning (ML) is a subset of artificial intelligence (AI), which provides systems the ability to automatically learn and improve from experience without being explicitly programmed.
Machine Learning Algorithms play a crucial role in the context of IDR specifically in the efficient extraction of data and document classification. IDR systems use supervised and unsupervised learning methods to process large volumes of documents. Through supervised learning, algorithms are trained on a pre-labeled dataset to recognize and categorize different document types and their respective contents. This training involves providing the algorithm with examples of documents and their corresponding classifications so it can learn and apply this knowledge to new, unseen documents.
In unsupervised learning, the algorithm tries to identify inherent patterns and structures in the data without pre-labeled examples. This can be particularly useful for clustering similar documents together based on the content and layout. This allows organizations to organize and process documents even when there’s no initial training data available.
One of the key advantages of using machine learning in IDR is its ability to handle a wide variety of document formats and types. Algorithms can be designed to be adaptive, improving their accuracy and efficiency as they process more documents. This is particularly beneficial since documents, such as invoices, contracts, and identification papers, may come in different formats and designs.
Another way that ML contributes to IDR is through feature extraction. Algorithms can analyze and pick out key features from texts, such as specific words, phrases, dates, or numerical data, that are important for determining the context and relevance of the information. This functionality is especially important for categorizing and extracting specific data points from complex documents reliably.
Moreover, the ability of machine learning algorithms to continuously learn and adapt means that the IDR systems can become more accurate and efficient over time. This increases the quality of data extraction and decreases the likelihood of human error, which is crucial in high-stakes environments like legal, banking, and healthcare where the correctness of extracted data is paramount.
In document classification, ML algorithms are trained to recognize the structural and textual features of certain document types. For example, a trained model can distinguish between a legal contract and a technical manual based on word choice, document layout, and other distinguishing features. As these algorithms process more documents and receive corrective feedback, their classification accuracy improves.
Overall, Machine Learning Algorithms are essential for the development of effective IDR systems, enabling the automation of data extraction and document classification processes, reducing the time and labor costs associated with these tasks, and increasing the overall accuracy and efficiency of data management systems.
Natural Language Processing (NLP) Techniques
Natural Language Processing (NLP) Techniques are a set of computational methods that enable computers to understand, interpret, and generate human language. NLP sits at the intersection of computer science, artificial intelligence, and linguistics. Its goal is to bridge the gap between human communication and computer understanding. At the core of NLP are algorithms designed to process and analyze large amounts of natural language data. This involves various tasks such as speech recognition, natural language understanding, natural language generation, and sentiment analysis.
One of the primary applications of NLP techniques is in the field of information retrieval and data extraction, where they are used to pull specific information from large documents or datasets. NLP can identify and extract named entities (such as names of people, organizations, locations), specific facts, dates, figures, and relationships from texts. This capability is crucial for industries like law, healthcare, and finance, where the ability to quickly find relevant information can greatly impact decision-making and operational efficiency.
When it comes to document classification, NLP plays a critical role in automating the process. By understanding the context and meaning of the text, NLP techniques can classify documents into predefined categories based on their content. This automated classification saves time and reduces the need for manual intervention, allowing for more efficient information management.
Intelligent Document Recognition (IDR) technologies leverage NLP to automatically recognize, categorize, and route information contained within various types of documents. By recognizing the context and semantics of words and phrases within documents, IDR systems can go beyond basic pattern recognition and keyword matching. They can discern the purpose of a document, differentiate between document types (e.g., invoices, contracts, reports), and extract relevant information with high precision.
For efficient data extraction and document classification, IDR systems use NLP to:
– **Improve Precision**: Where simple pattern matching might fail due to variations in language or document formatting, NLP can understand the underlying meaning and extract information with greater accuracy.
– **Handle Complexity**: NLP can work with complex sentence structures, idioms, and nuances of human language, making sense of unstructured data that would otherwise be inaccessible to computers.
– **Enable Contextual Analysis**: By understanding context, NLP-powered IDR systems can discern the relevance of particular fragments of text, making it possible to identify the most important parts of documents for extraction.
– **Adapt to Changes**: NLP models can learn and adapt to new patterns in language usage over time, making IDR systems more resilient to changes in how information is presented in documents.
In summary, Natural Language Processing (NLP) Techniques contribute significantly to efficient data extraction and document classification within IDR systems by providing a deeper understanding of language, which enables a more nuanced and accurate interaction with text data. These advancements help organizations manage and utilize their vast amounts of unstructured data more effectively, enhancing their productivity and decision-making capabilities.
Optical Character Recognition (OCR) Integration
Optical Character Recognition, commonly known as OCR, is a crucial technology that converts different types of documents, such as scanned paper documents, PDFs, or images captured by a digital camera, into editable and searchable data. OCR integration is item 4 on the provided numbered list, and it is essential to the process of intelligent data recognition (IDR), which encompasses efficient data extraction and document classification.
OCR plays an integral role in IDR by accurately scanning text within images and converting it to a digital format that can be searched, analyzed, and processed by other computerized systems. This conversion is the first step in data extraction, where information is taken from a document and made available for further processing. Traditional OCR technology required structured forms and data for it to function accurately. However, modern OCR is much more sophisticated and can handle unstructured data with increasing accuracy, thanks to advances in machine learning and artificial intelligence.
Once documents are digitized, the text can be used in conjunction with other technologies listed in the enumerated list, such as advanced pattern recognition and natural language processing techniques, to further enhance the accuracy of data extraction. For instance, OCR technology will convert the image of the text into raw data. Afterwards, pattern recognition can be used to identify and categorize specific data patterns within the text, while machine learning algorithms can be fine-tuned to continually improve the recognition process based on new data.
In terms of document classification, the integration of OCR allows businesses and organizations to automate the sorting and organization of vast amounts of documents. By recognizing text and patterns within documents, OCR-enabled systems can categorize and tag documents based on their content, thus facilitating quick retrieval and improving workflow efficiencies. This can be particularly beneficial in industries where there is a high volume of paper-based documentation, such as law, healthcare, and finance.
Moreover, the combination of OCR with intelligent systems utilizing machine learning algorithms enables self-improving classification models. As these systems are exposed to a larger variety of document formats and text layouts, they learn to better recognize and classify incoming data. This not only increases the accuracy of document classification but also reduces manual intervention and processing time.
Overall, OCR integration within IDR solutions provides a critical stepping stone from the analog to the digital world, allowing for the unlocking of textual information in static documents and images. When applied in conjunction with other intelligent technologies, it significantly enhances the capability for efficient data extraction and document classification, driving productivity and reducing costs in information-driven sectors.
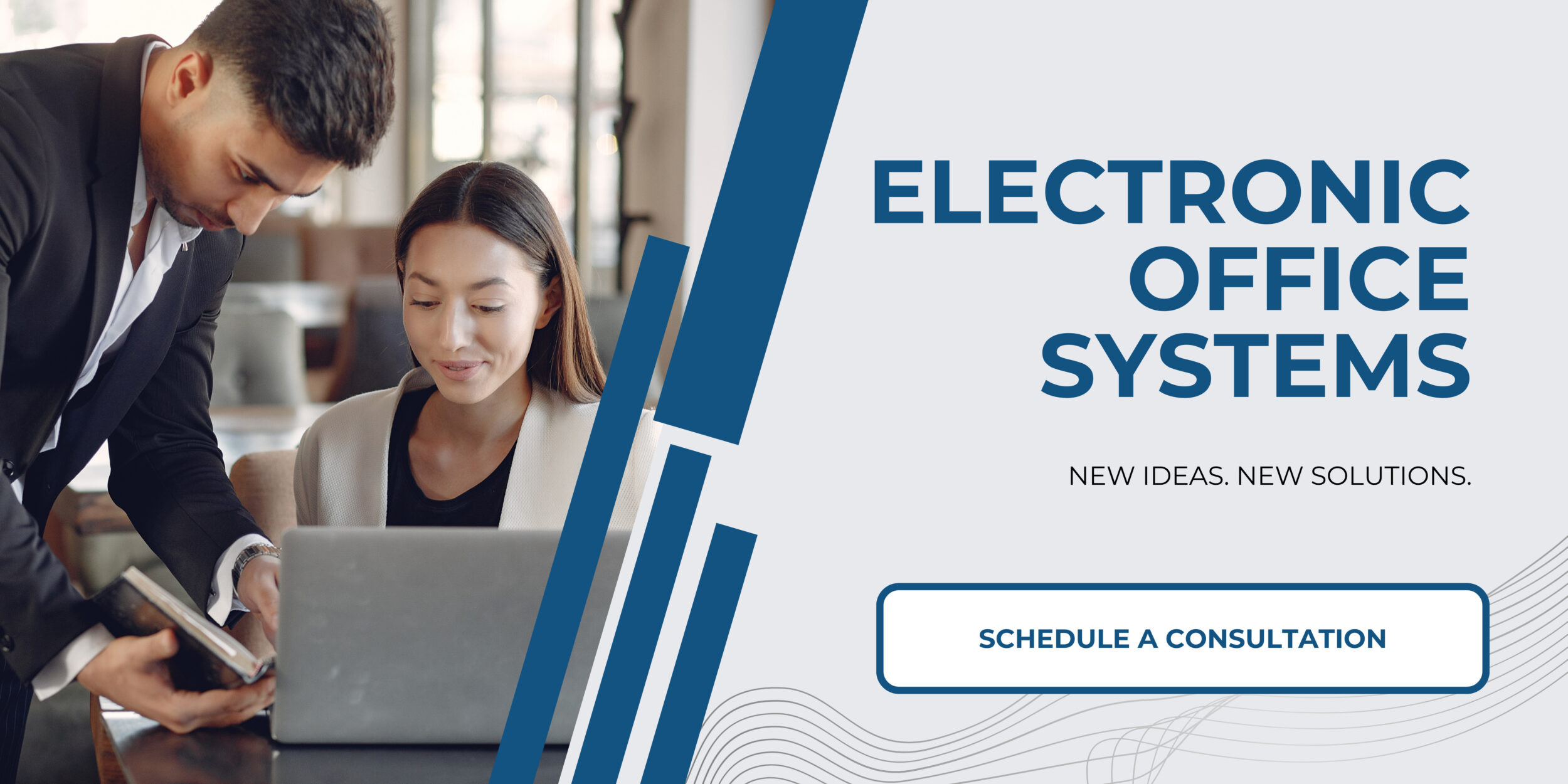
Scalability and Adaptability for Diverse Data Sets
Scalability and adaptability are crucial characteristics for any system that’s designed to handle data, and that includes Intelligent Document Recognition (IDR) systems. Growing businesses and evolving sectors generate increasing volumes of data, and such data can significantly differ in structure, format, and content. A system that can scale means that it can handle growing amounts of data—ranging from dozens to millions of documents—without a corresponding increase in processing time or a decrease in performance. The adaptability aspect refers to a system’s capacity to process and understand various types of data sets that may be structured or unstructured, come in different languages, or contain unique formats, without requiring an entire system overhaul.
IDR’s contribution to efficient data extraction and document classification lies in its ability to process large and diverse data sets with high accuracy. Traditional data extraction methods required extensive manual work, were prone to errors and couldn’t easily manage the increasing complexity and volume of today’s data. IDR systems use advanced technologies like Machine Learning (ML), Natural Language Processing (NLP), and OCR to adaptively learn from the data they process. This fosters an environment where the system can improve over time, recognizing patterns and structures within documents regardless of variances in datasets.
The scalability of IDR systems means that as a business or organization grows, the data handling capacity grows with it. There is no need to invest in new processing capacities or radically change the system’s infrastructure. Instead, an IDR system can be designed to grow incrementally to match the increase in demand. This can represent significant cost savings and efficiency gains as there is less need for the processing overhead that comes with manual systems.
Adaptability also extends to the handling of different types of documents. Whether they are invoices, receipts, contracts, medical records, or any other types of paperwork, adaptable IDR systems can classify and extract data from these documents effectively. This capacity is vitally important in fields like healthcare, finance, and legal sectors where documents may contain complex and specialized information.
Through the use of sophisticated algorithms, IDR systems can learn to differentiate important data points within a diverse range of documents and disregard the irrelevant details, which significantly streamlines the data extraction and classification processes. The system’s ability to adapt to new or changing document types or data structures without requiring extensive programmatic changes is a testament to its flexibility.
By pairing scalability and adaptability, IDR systems provide a robust solution for organizations to manage their data extraction and document classification challenges. As IDR technology continues to evolve, it’s likely that these systems will become even more adept at handling the complexities of a world increasingly driven by data.